How to increase your AI product stickiness with Phospho
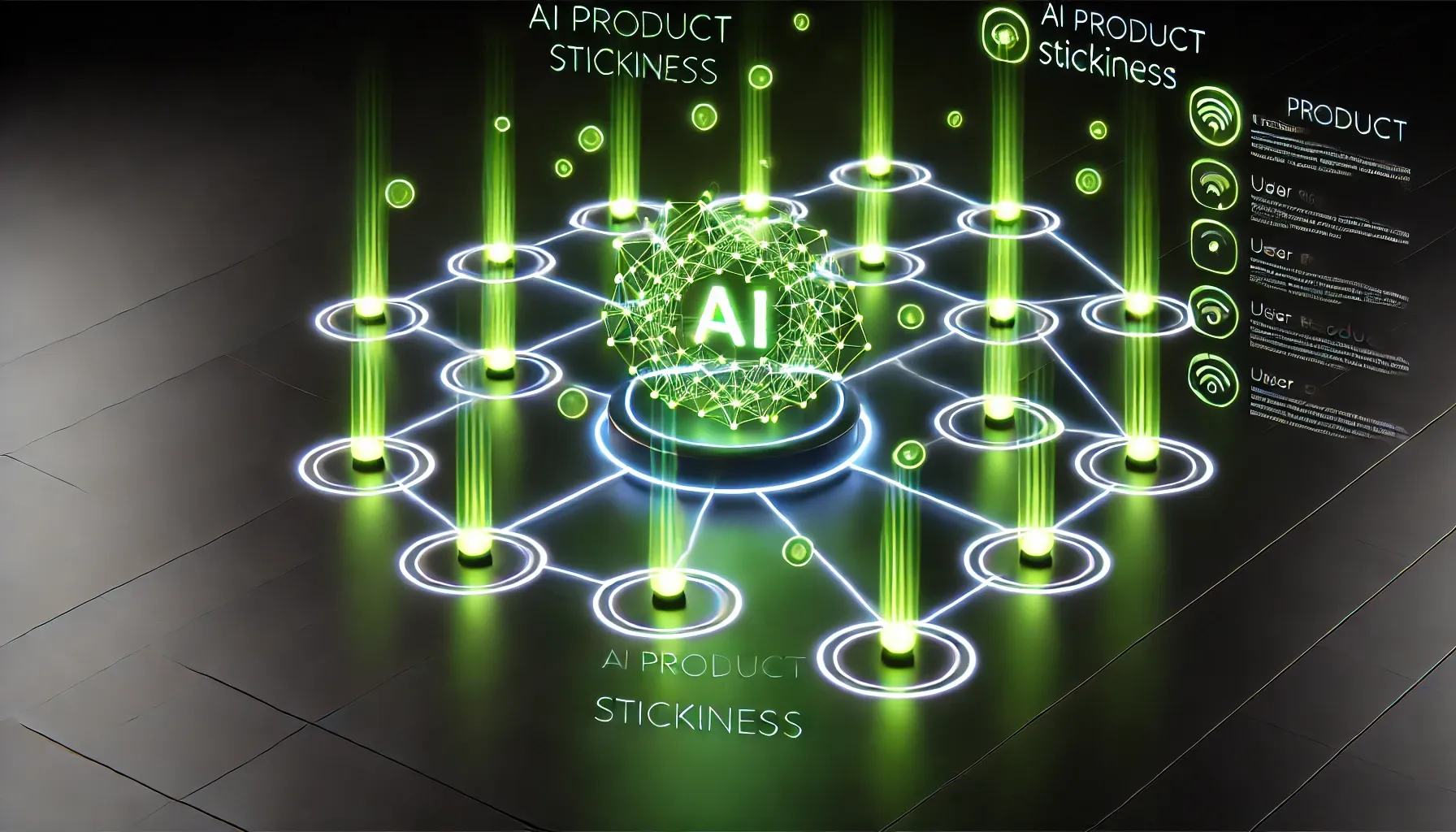
Crazy news guys, we’ve just launched a startup program for AI founders (the perks are crazy).
You can get $2000 worth of credits (Anthropic, Mistral, OpenAI, and Phospho) + a call with our amazing team to guide you in your product-market-fit journey.
You can apply here.
Product stickiness isn’t just how often users return, it refers to how frequently users open your app, engage with it, leave and then come back. This is why you’ll likely find many different definitions of it online if you have a quick Google.
It’s often measured by the daily active users (DAU) to monthly active users (MAU) ratio, which was popularised by Facebook. So, for example, a product with a DAU/MAU of 50% means users use it every other day in a month. The average stickiness rate for a SaaS is 13%, and it is generally considered anything above 20% is good.
Stickiness is an extremely popular engagement metric. Product managers use it to indicate a product’s ability to engage and retain users to inform roadmap decisions, and investors adore it for helping them determine investment worthiness.
In this article, we’ll examine product stickiness, specifically for AI products, which have seen an exponential rise in development since integrations with LLMs like Claude and Chat GPT have become easy.
Understanding the AI Product Landscape
The rise and rapid adoption of AI has vastly accelerated the production of AI products. With more apps integrating AI LLMs into their tech stack, these 'novels’ features leveraging AI are not best practices (yet) and propose new challenges around retention.
With a staggering 85% of AI initiatives failing to deliver the expected ROI, the pressure is on to demonstrate technological prowess and real, tangible value. Without years of proven best practice insights like you would get for traditional features, the focus for AI integrations is now on feature improvement instead of feature optimization to improve retention and stickiness.
What’s the difference between feature improvement and optimization?
Feature improvement is a phase focused on adding more value, whereas feature optimization aims to optimize the product itself.
To increase the value of an LLM app you need to increase product stickiness, and for that you need to understand as well as MEASURE the way users interact with it.
Importance of measuring stickiness
Measuring and tracking product stickiness is what develops a clear picture of how your product performs over time. Whenever we go through iteration cycles and update our AI product, we’ll need that clarity in knowing if we’re making the right choices and investments into our development roadmap.
As customer stickiness increases, your churn will naturally decrease. Decreasing churn is a critical component of bringing down customer acquisition costs while increasing your customer base, thereby freeing up cash and accelerating growth. Measuring and optimizing stickiness addresses churn head-on.
Stickiness should also factor into your upsell strategy. If you attempt to upsell to customers who don’t find value in your current product, at best, your upsell efforts may be wasted; at worst, you risk alienating already unhappy customers.
Earlier in the article, we mentioned how the DAU/MAU ratio popularly measures stickiness. Unfortunately, though, it’s not that simple. Relying only on this ratio to see if our product is sticky has real limitations, as it doesn’t paint the full picture.
For example, the ratio doesn’t account for the total number of users, which can be problematic because it will inevitably lead us to the wrong conclusions. For instance, you can get the same DAU/MAU ratio from a lot of usage over a short period, or from short consistent usage over a longer time.
It's important to look at stickiness over different times to see what's going on with your users and what works best. This metric should be considered alongside other data points to provide the insights necessary to make informed product decisions or evaluate your product’s stickiness.
So how do we actually measure and improve the stickiness of an AI product?
How to improve AI product stickiness
Numbers and ratios aside for a minute, stickiness is really a testament to your product’s ability to fulfill user needs and maintain a lasting relationship with them. Sorry for anyone reading that likes their concrete numbers.
So instead of a broad sweeping definition, let’s contextualize the above statement into strategies we can actually use to directly improve the stickiness of our AI products:
- Personalized onboarding experiencesA clear and enjoyable onboarding process can help your new customers better understand your product and its value. It minimises confusion, dissatisfaction, or the lack of perceived value - all factors that can lead to abandonment.For example, WeMoney, an Australian wellness company, noticed that users who engaged with its goal-setting feature tended to stick around more. But the goal-setting feature wasn’t easy for WeMoney users to find. By building the goal-setting feature into its onboarding process, the company saw a 20% boost in users sticking with the product.Design your onboarding flow to be simple. Guide users through key functionalities, minimise the number of steps and avoid overwhelming them with unnecessary information.
- Personalize features or contentUse customer data to create a more personalized and engaging experience that encourages users to stay connected with the product. Content, recommendations, and features specifically relevant to your users’ preferences, behaviors, and needs will help drive that personalization.By giving users content that aligns with their interests or past interactions, you can increase the likelihood they’ll continue engaging. In fact, 57% of consumers in Twilio’s State of Customer Engagement Report said they’d spend more with a brand that personalizes their experiences.By using AI product analytics tools such as Phospho for LLM apps, you can obtain metrics such as user behavior patterns and interactions in real-time to give yourself—or any product team, for that matter—valuable insights into individual user preferences. You can then use this data to create tailored improvements that really form better connections with your users and keep them coming back.
- Continuous feature updatesShowing users you understand them can be tricky but by improving features based on feedback, trends, and preferences it shows that you are actively ‘listening’ to their wants and needs.Analysing data from your users is listening to what they’re saying, it’s not always in a literal sense. Then, keep improving your product offerings and testing new features based on those observable metrics. This feedback loop keeps your product fresh and aligned with what users love, making them more likely to ‘stick’ around.
It comes as no surprise then that the two kingpins of iterative development rear their heads again as critically important components to understand:
- Feedback
- User behavior
We need both to iterate in line with what your users really want - this is what ultimately keeps them coming back.
For that we need tools, specifically, tools that let us deeply understand those 2 crucial components. Thankfully, they exist today in tools like Phospho which employ text analytics to derive insights from the conversations your users share in your LLM app.
So if you’re creating an LLM app and want to gain untapped insights from your text data, sign up here!
Future of AI product stickiness with Phospho
Data is a key component in creating a sticky product. Quantitative data helps you understand customer preferences, trends, and patterns so you can give users a valuable product they can incorporate into their lives. For example, using product benchmark metrics help you measure your product's performance against other products in your industry.
Start leveraging data by implementing robust analytics tools and methodologies. If you’re unsure where to start, Phospho offers a suite of features that allows anyone developing AI LLM apps to analyze data effectively.
Phospho is an open source text analytics platform designed for LLM apps to monitor, learn and evaluate text data to drive effective iteration. Here are some features we’ve specifically developed to help you derive rich customer insights to tailor products to customer needs and preferences so you can drive long-term engagement and growth:
- Real-Time Monitoring: this lets you track and log user inputs to identify issues or trends as well as continuously fine tune the performance of your LLM app.
- Custom KPIs Extraction: Create your own KPIs and custom criteria to ‘flag’ for, and you can label whether it was a successful or unsuccessful interaction.
- Continuous Evaluation: use our automatic continuous evaluation pipeline to keep improving your model’s performance.
- Easy Integration: simply add Phospho to your tech stack with any popular tools and languages like JavaScript, Python, CSV, OpenAI, LangChain, and Mistral.
- User Feedback Linking: collect, attach, and analyze user feedback in context to make targeted improvements toward overall app performance.
If you want to confidently iterate according to user needs and create more efficient, accurate, and user-centric LLM apps, sign up here and try out Phospho on your own data.