Claude 3 Opus Context Window: How to Use it For Your LLM App
Claude 3 opus context window transforms LLM apps by enhancing customer support, personalized content creation, and advanced data analysis, ensuring better accuracy and context retention.
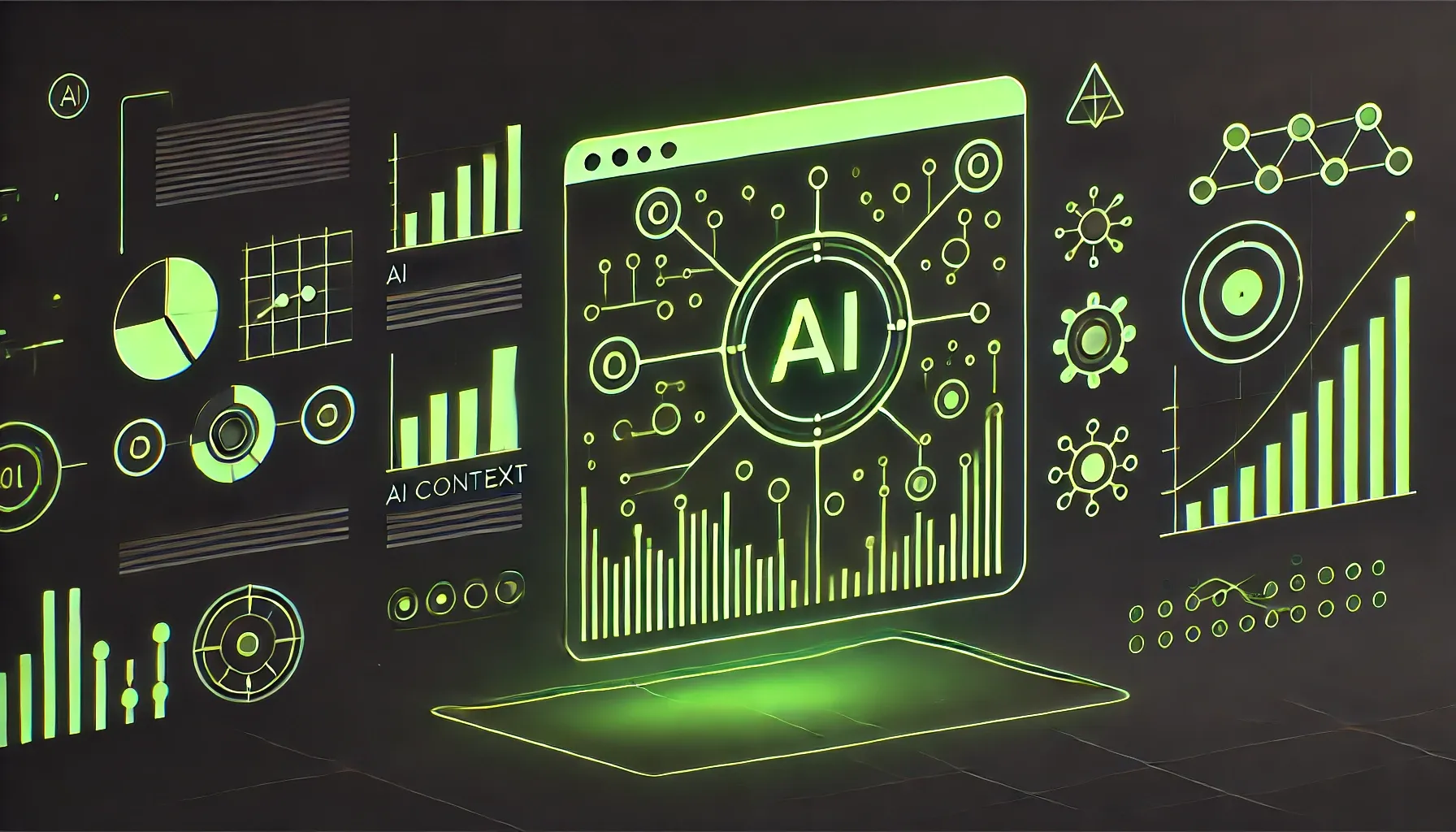
Exciting news, phospho is now bringing brains to robots!
With phosphobot, you can control robots, collect data, fine-tune robotics AI models, and deploy them in real-time.
Check it out here: robots.phospho.ai.
An LLM app is any application with a built-in feature(s) utilizing advanced AI models, (EG: Claude) that have been trained on huge amounts of data.
An app description like that suggests they have wide-reaching applications across industries, and it does…
The ability for these apps to understand and generate human like text has seen them deployed for:
- Customer service
- Content creation
- Software development
- Legal & Compliance
The list goes on but a key feature to LLM apps is their context window - the most amount of text the model can use and consider when generating responses. In other words, their memory.
Common sense dictates then that larger context windows allow for more persistence of context and coherence over longer conversations i.e a better LLM app.
Most recently, Claude 3 has recently released a significantly expanded context window, which opens the door for more sophisticated and context aware apps with far better accuracy and relevance in responses.
We don’t need to convince anyone the value this text data holds for streamlining iteration cycles, and it’s exactly why we’ve built our text analytics platform Phospho for.
If you want to leverage the insights available to you in your LLM app, sign up here and get started with your own test data!
If you'd like to learn more about AI software product engineering check the details guide we wrote here
We've also built a free guide on how to build AI SaaS in 2024 with a data-driven approach. You can get access: Here
If you want to learn how to build actionable AI dashboards you can also check this free guide: Here.
3 specific use cases for claude’s context window
There is no shortage of options in today’s LLM landscape, but Claude 3 stands out for its better understanding of complex queries and the nuance of human communication.
To understand why we must first define context saturation which refers to the point the context window is full and reduces the ability to utilise earlier context.
This means that the more tokens a context window has, the more context you can feed before saturation and degradation occur in responses.
For comparative understanding, the earlier models like Chat GPT-3 had context windows of around 2048 tokens and jumped up to 32,000 with the GPT-4 model.
Claude however boasts a much larger context window of 200,000 tokens which helps provide far more accurate responses, retention of deeper context and reduced hallucinations.
Use Case 1: Customer Support
Example: you’re a SaaS startup and a customer needs to contact support about a specific issue with the product.
With a larger context window, we can retain a larger memory of the customer’s data, such as their full purchase and support history or any previous troubleshooting attempts.
This is important because it also means we can leverage deeper product knowledge for more accurate support and consider a wider array of contextual factors to provide a more holistic solution.
You could leverage text analytics to:
- Monitor the customer’s conversation and track the accuracy and relevance of Claude 3’s responses
- Analyse customer sentiment during support handled by Claude
- Forecast issues from patterns that will require human involvement (proactive approach)
With more bandwidth in Claude 3 opus context window and continuous improvement using text analytics, we can provide much better customer support and a side step becoming a generic chatbot that regurgitates website information.
Use Case 2: Personalised Content Creation
Example: you’re a micro-education platform that provides personalized modules for learning quickly.
Personalization will have a linear correlation with an LLM app’s ability to retain the context of a user’s data, such as:
- Persona (title, industry and interested subjects)
- Areas of difficulty
- Usage patterns
- Support interactions
Progressive understanding of the user’s needs in real time is a game changer for offering personalised tips and courses for learning more effectively and actually providing what the user needs.
You could leverage text analytics to:
- Refine course delivery in line with patterns in user behavior and preferences
- Visibility in whether course delivery methods are engaging and effective through A/B testing and evaluation
Use Case 3: Advanced Data Analysis
Advanced data analysis doesn’t pain enough of a picture so imagine the example below:
You’re an e-commerce brand that needs to optimize your marketing strategy based on customer behavior.
When trying to sell our products, we need to make sure our marketing efforts reach the right people and resonate with them.
A larger context window would allow more customer data ingestion without losing wider context.
Using this data to understand purchasing habits, trends, and preferences can fuel the testing of different marketing strategies from a data-driven standpoint.
This would help us identify what’s working and what’s not with different customer demographics and allow for more personalized marketing strategies with effective messaging and timing.
Leverage text analytics to:
- Track sentiment for personalized marketing (optimize conversion)
- Measure the effectiveness of new campaigns and testing
- Identify patterns to forecast buying intent
The above use cases barely scratch the surface of what AI and LLMs can be capable of today, let alone in six months.
Claude’s expanded context window signals an opportunity for far more context aware LLM apps across industries.
But companies will need to adopt advanced text analytics tools like Phospho to fully leverage the insights available in their users’ interactions with LLM apps.
Claude 3: A No Brainer for LLM Apps?
The AI industry is evolving rapidly, but anyone can get ahead with the right tools.
At Phospho, we aim to provide these tools and the leverage to gain rich insights from LLM apps.
Here’s how you can get started:
- Create your Phospho account
- Import your data in a project (as easy as Excel or CSV)
- Set up events and get insights on your dashboard
It’s that simple to streamline your insights gathering and iteration cycle.
Want to take AI to the next level?
At Phospho, we give brains to robots. We let you power any robot with advanced AI – control, collect data, fine-tune, and deploy seamlessly.
New to robotics? Start with our dev kit.
👉 Explore at robots.phospho.ai.