The Future of Ad Hoc Analysis in AI Product Development: How to use Phospho for Insightful AI-data analysis
Ad hoc analysis is essential in AI product development for quick, data-driven decisions. Phospho offers real-time insights, enabling rapid iteration and optimal AI model performance, keeping you ahead in a competitive market.
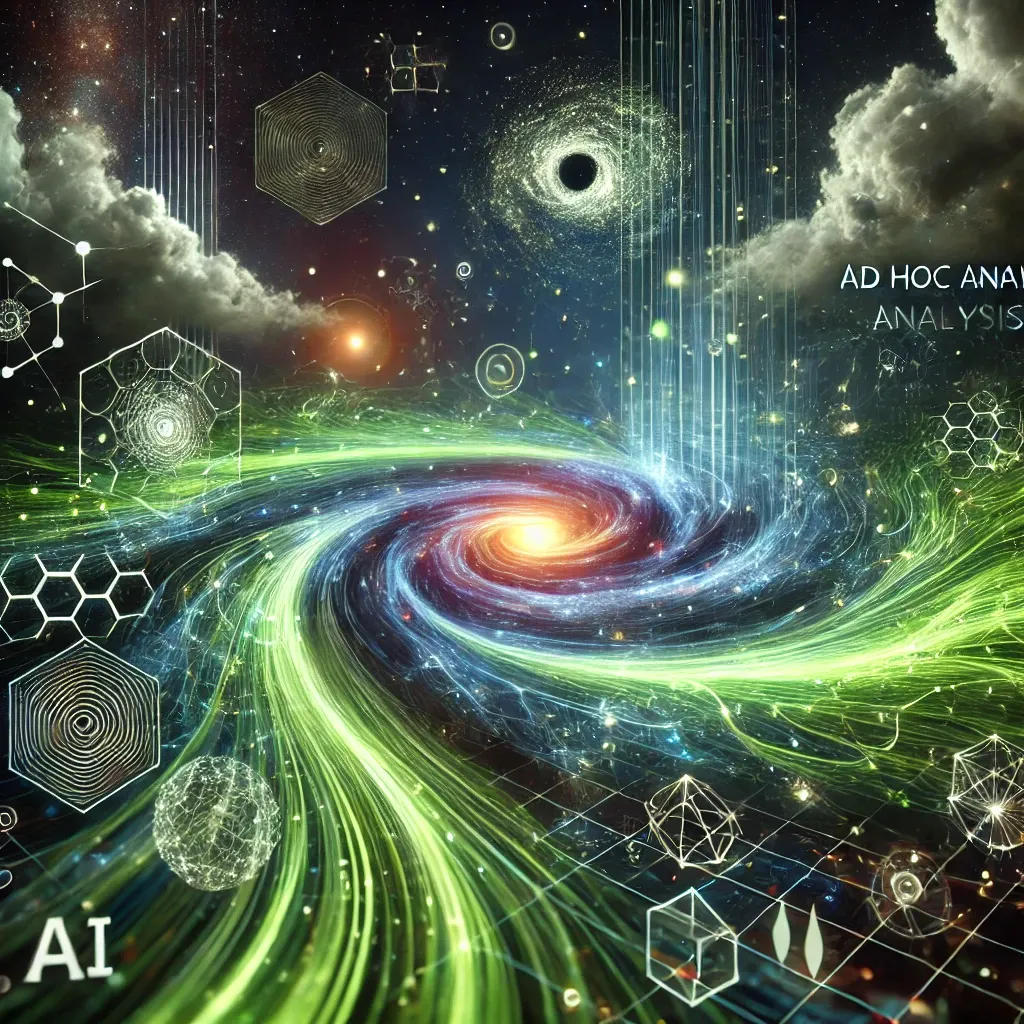
Crazy news guys, we’ve just launched a startup program for AI founders (the perks are crazy).
You can get $2000 worth of credits (Anthropic, Mistral, OpenAI, and Phospho) + a call with our amazing team to guide you in your product-market-fit journey.
You can apply here.
Ad hoc analysis is basically a form of data exploration where you ask specific queries to gain insights rather than waiting for reports or dashboards. It suits environments where data changes frequently and product iterations are needed quick and fast.
Therefore, it’s particularly applicable in the world of AI product development where the speed at which you can make informed product decisions relies on whether you can obtain immediate and actionable insights from large amounts of data constantly subject to change.
With competing AI products becoming more sophisticated as well, the ability to pivot quickly based on new data, feedback, and pattern changes can largely determine the success of your product in the market.
Traditional methods of analysis often rely too much on static metrics or pre-defined KPIs which struggle to capture the nuances, unexpected trends, or emerging edge cases that often come with AI product development.
This lack of data clarity is important to address with more capable, modern tools because it can lead to missed opportunities and slow decision making which cripples your competitiveness.
For AI product development today, we need advanced analytics tools specifically made for AI products. One of those available to AI product teams is our open source analytics platform, Phospho.
We noticed the lack of tools catered for AI products so we built Phospho specifically for this reason. This way, modern product teams can easily monitor, learn, and evaluate their AI products in real-time to provide rich, actionable insights. You can try Phospho for free by signing up here.
If you want to see more of Phospho’s features and use cases first to see if it’s a good fit for you, read our previous article here where we compare Phospho with different product analytics tools available to product teams right now.
Ad Hoc Analysis in AI Product Development
Unlike regular analysis and reporting, ad hoc analysis is conducted on an as-needed basis to provide immediate insights into particular queries or issues.
It’s role in the AI product development lifecycle is critical for rapid, well informed iteration. The ability to instantly query and analyse data related to model performance, training, and post-deployment monitoring is vital for products with AI outputs that need constant refinement and fine tuning.
Let’s take a common occurrence as an example: there’s a sudden shift in user behaviour after an update and you don’t know why.
Immediacy is extremely high value when coming up against unanticipated challenges. The quicker we understand them and address them, the more risk we can mitigate.
Instead of waiting for batch processing or aggregated reporting systems, with real-time exploration you can immediately ask queries to narrow down the reasons why and address any implications this may have on specific feature or AI model performance.
Phospho has capabilities built in so you can handle situations like these effectively. We’ll go into our features more in the next section, but having tools like Phospho at your disposal for quick custom queries and real-time insights are what minimises the time spent addressing unanticipated problems so your AI models are performing optimally.
Phospho's Role in Modernizing Ad Hoc Analysis
To understand the role Phospho plays in ad hoc analysis, let’s take a use case to showcase its main features in action.
A hypothetical case could involve your AI model suddenly misinterpreting user intents after an update, which leads to user satisfaction plummeting, and you need to know why this happened.
Phospho’s real-time logging feature ensures that every user interaction and AI model output is captured and always available for immediate analysis. By looking through historic interactions individually you gain visibility into context and specific outputs that users were unhappy with. You can then annotate and contextualise individual data points that link directly to user feedback.
This minimises the time between user interaction and insight extraction which is a game changer for data driven iterations and efficiently addressing any issues.
But let’s take this a step further, Phospho allows teams to create their own custom KPIs to automatically track and ‘flag’ different interactions for.
In the context of our hypothetical case, after a problematic update you might want to track something specific for misinterpretation such as:
- the frequency of incorrect sentiment analysis during customer service interactions about refunds
- Or the accuracy of your model’s ability to differentiate between literal and figurative language for creative writing prompts
- the rate of misclassification in identifying urgent vs non urgent requests in chatbots
What we’re trying to showcase here is the flexibility and control you have with our features when obtaining relevant data for any scenario, edge cases, or unanticipated issues. This firmly supports the ad hoc analysis approach where you have freedom and accuracy in which to identify root causes and test fixes as and when you need.
You can also automatically A/B test any potential fixes to see which versions of your product perform best, ensuring your AI product is always optimising and improving.
The real benefit here is you can do all of this without any downtime or halting the development process so you can achieve much faster, better informed iteration.
If we want these capabilities, we’ll need to plug Phospho into our workflow. One if it’s key advantages is the ease at which you integrate with tech stacks and AI tools, without needing any changes. Simply add Phospho to your tech stack with any popular tools and languages like JavaScript, Python, CSV, OpenAI, LangChain, and Mistral.
Sign up here and try out Phospho on your own data. Even though our integrations are seamless, we have plenty of supporting documentation as well.
Advantages of Using Phospho for Ad Hoc Analysis
Modern AI product teams need to be able to answer queries without getting caught up in complex workflows or disjointed tools you have to stitch together. As AI product developers ourselves we deeply understand the need for a single source of truth that is simple, collaborative, and comprehensive.
So despite its powerful capabilities, we designed Phospho with accessibility and ease of use in mind. After seamlessly connecting Phospho, you can use its intuitive interface where even non technical team members can perform ad hoc analysis. Much like ourselves, we wanted to make sure access to insights was democratised for the entirety of any team to encourage wider collaboration that can help draw better conclusions from diverse perspectives.
Data will only continue to grow more important and teams will increasingly rely on its insights as a competitive advantage. But as AI products scale and generate larger volumes of data, we’ll need ways to ensure ad hoc analysis can remain as effective at early stage as it is when our companies grow in size and data complexity. Phospho’s architecture can handle increased loads and complexity without compromising on performance which ensures you can keep ad hoc analysis as a practical tool even as you scale.
In addition, it’s also a sensible adoption in terms of cost effectiveness against traditional third party tools. Our open source nature positions Phospho as an analytics platform you can tailor for your specific needs, saving both time and money.
Predicting Trends with Ad Hoc Analysis in Phospho
One of the best use cases for properly applying ad hoc analysis is the potential for staying ahead of potential issues and proactively addressing them. Access to real-time data and Ad hoc analysis in Phospho can help teams identify any emerging trends before they become obvious or problematic.
Let’s take another hypothetical example: an AI driven chatbot starts receiving an influx of complaints about a specific issue from a recent update.
Using Phospho to detect this trend early, AI product teams can investigate with ad hoc analysis to understand the root cause, and make proactive adjustments to their models’ responses by fine tuning classifiers or providing more training with new data as examples. This ensures your AI model can remain effective as different user needs and edge cases evolve with your product.
But we can be even more predictive with Phospho’s scenario planning. We enable teams to simulate various scenarios using real-time data to help you understand how different interactions might affect your model’s performance.
This is particularly useful for high stakes products where predictive accuracy for new updates is critical. From a macro perspective though, it provides any team with a significant competitive advantage where your AI model’s accuracy and relevance is a determining factor to success in a saturated and fast moving market.
The Future of Ad Hoc Analysis in AI
Ad hoc reporting’s query based analysis is an effective way to gain insights into your data that you would find difficult while using traditional analytics.
It’s clear to see that ad hoc analysis and AI analytics provide synergy, but more importantly, this synergy is critical to startups and scale-ups who need to establish competitive positioning fast. It’s the actionable insights we can derive from real-time AI analytics that provides the leverage for user aligned iteration and therefore faster product market fit.
To ensure your AI models are effective and stay ahead in the constantly evolving AI market, try Phospho’s open source AI analytics for free by signing up here.