The importance of AI product analytics in 2024
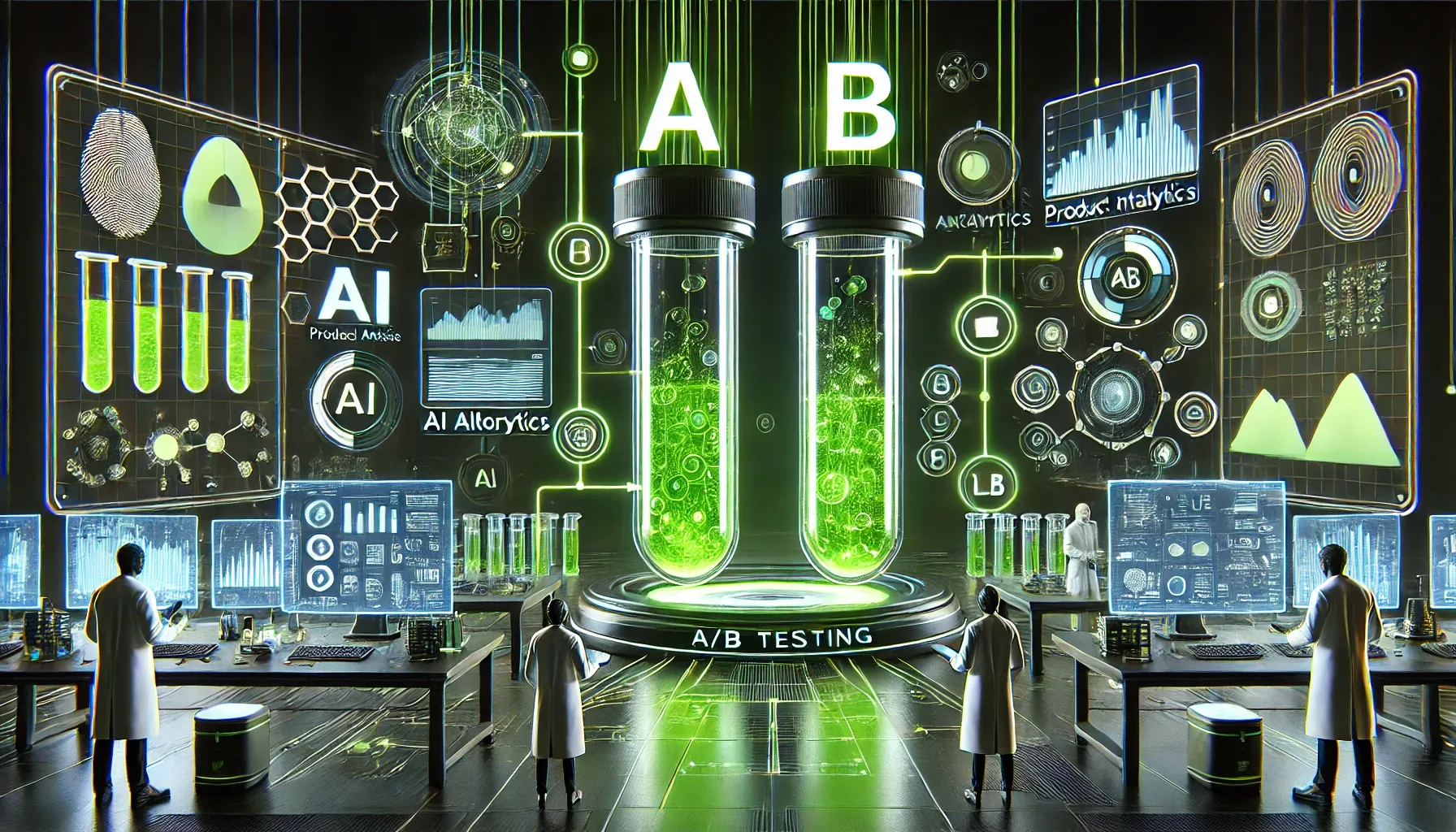
Crazy news guys, we’ve just launched a startup program for AI founders (the perks are crazy).
You can get $2000 worth of credits (Anthropic, Mistral, OpenAI, and Phospho) + a call with our amazing team to guide you in your product-market-fit journey.
You can apply here.
Everyone is talking about Gen AI these days and the massive data sets it can produce from being integrated into apps. With this rise in big data, AI product analytics is a no-brainer for your overall decision-making process. So, what exactly is it?
AI Product Analytics is the application of artificial intelligence to market and product data. Product Teams can use AI to automate data processes and analyze market and user data – including identifying patterns, delivering data insights, and making predictions of future trends.
But product analytics WITH AI means these systems learn and improve over time, making predictions more accurate. This lets businesses look at huge amounts of data, giving deep insights that old methods simply won’t ever match. For example, over 80% of Netflix content is found through AI-powered recommendations.
Why should we monitor AI applications?
Data is the new gold and has been for quite some time. But obtaining data is what helps SaaS companies make more informed decisions and ultimately better guide the evolution of the product.
Creating data-driven teams at every level is one of the most significant trends and imperatives in the AI startup space to not only build better products but to remain competitive against the rest of the market.
In the same way that no-code tools empowered non-technical teams to build and freed up engineering capacity, AI analytics will give everyone in the Product Team a key to peek into the black box of data. In fact, they can do more than peek in; they can walk away with armloads of insights.
This is the promise of AI product analytics– not to replace product managers, but to set entire product teams up for success. With the support of AI analytics platforms like Phospho, you’ll have data to help you correctly identify customer problems, prioritize initiatives, and streamline iteration cycles.
If you’re creating an LLM app and want to gain untapped insights from your text data, sign up here!
4 KPIs for evaluating AI models with Phospho
KPIs are used to help measure success and improve things about your product and measuring the success of your AI model’s iterations is critical for several reasons. Firstly, it allows you to determine whether new implementations are meeting their intended goals, such as improving efficiency, increasing sales, or raising customer satisfaction.
Without concrete metrics, it’s difficult to clearly determine the actual tangible value your updates are adding and to make informed decisions about future versions of your AI product.
Let's examine the main KPIs for AI products and how Phospho can be used to monitor them in real time and provide actionable insights.
1) User interaction metrics
These metrics measure users' interaction and involvement with a product. They provide insights into how actively users engage with content, features, and functionalities and can help you assess the effectiveness of your AI model’s ability to capture and retain user attention.
By monitoring this KPI your AI model can be fine tuned to provide responses that are more relevant and engaging which would directly lead to more user satisfaction. Companies like Lyft use AI to make user interactions better by matching driver supply with customer demand in real time.
Phospho logs user interactions in real-time, providing detailed analytics on engagement patterns. For example, by defining what is a successful and unsuccessful response based on engagement patterns can fine tune your AI model’s responses to provide more accurate and relevant responses your users actually want while using your LLM app.
2) Error rate metrics
This metric is for checking the accuracy of AI outputs. Probably one of the most obvious reasons for implementing AI would be in admin heavy processes such as data entry, calculations, and transaction processing. Not only to save time but in particular to reduce human error.
The error rates may be tracked before and after the implementation of AI to underline in which areas improvement in accuracy is really needed.
This should not clear the radar from view: the fact that error rates are expected to be monitored continuously, since LLM performance can drift over time due to changes in usage patterns or updates to the underlying model. In these regards, to ensure the quality and reliability of your AI product with respect to LLM integrations, you should have its error rate regularly monitored and analyzed.
Phospho lets you do exactly that by logging user inputs and LLM outputs for continuous fine-tuning of the performance of your LLM app and the accuracy in its responses. Phospho also supports A/B testing of different versions of your LLM app so that you will be able to analyze the performance and accuracy of various iterations. You will be able to see what changes bring improvements and what does not; hence, this will provide the possibility of data-driven decision making at a time when you are refining your AI model.
3) Response time metric
Response time metrics become important, particularly when integrated into chatbots or conversation interfaces where the interaction is effectively a 'live' chat.
Faster response times in customer service lead to higher levels of customer satisfaction. AI helps minimize these response times not only by sending automated responses but also by providing the human operator with faster data retrieval and decision support.
Phospho can be leveraged in this case to associate the variance in response times to certain trigger events. For example, identifying bottlenecks by pinpointing specific queries or scenarios that produce longer response times can help teams focus their iteration efforts and optimisation on hyper targeted problem areas of their LLM app.
You can also set up custom events for tracking specific response time thresholds to produce alerts when acceptable latencies are exceeded.
By using Phospho to monitor your LLM app’s performance in real time you can concretely correlate response times with user satisfaction rates for further fine tuning of your AI model.
4) Custom KPI metrics
Custom KPI metrics are made for specific context, use cases and business goals. They’re meant to ensure that the AI model’s performance is evaluated in terms of its actual contribution to the overall success of the business.
Using Phospho you can define tailored metrics KPIs to track specific aspects of your AI model's performance, such as the KPIs above: response accuracy, latency, and user engagement.
The scope to be able to add completely custom KPIs with Phospho allows for far deeper insights into your AI model’s performance, beyond surface level metrics. This means we can now facilitate far more targeted improvements in the areas both your users really need and the ones that drive the most success for your LLM app.
By tracking these essential KPIs, you can not only monitor the immediate benefits of different versions of your LLM app with A/B testing, but also strategically refine approaches to maximise long-term gains.
These metrics provide critical insights that help stakeholders understand the value your AI product is adding, thereby also supporting continued investment.
Examples of effective AI product analytics
AI has largely changed how we look at product analytics across many industries so let's see how various startups and some of your favourite apps have used AI to make their products better.
1) How Spotify improved user engagement
Spotify, as we all know, is one of the leaders in music streaming worldwide but are also famous for its popular playlist recommendations such as Discover Weekly and Daily Mix. This feature, however, had actually started off from a challenge they experienced:
With literally millions of tracks on the platform, Spotify had to find a way that would let users easily discover new music that suits their specific tastes and moods.
Spotify applied AI algorithms to analyze user listening habits and taste in music, among other contextual factors. AI-powered music recommendation changed how users discover and engage with music on Spotify. This is because it generates millions of individually tailored playlists every week to stimulate more user activity.
These algorithms use AI product analytics to continuously tune recommendations based on user engagement and occasional feedback.
AI product analytics have basically caused huge user interest for Spotify. They now report that over 40% of its users are listening to AI-made playlists regularly.
2) How Abridge reduced error rates in Healthcare
Abridge is a healthcare AI startup that claims to help providers save an average of 2 hours per day on documentation simply from being able to quickly trust and verify AI generated recommendations for clinical practitioners.
By implementing “Linked Evidence” technology, Abridge was able to map AI-generated text to grounded truth, which provides far more confidence in AI-generated recommendations. By improving the accuracy of AI-generated clinical notes, Abridge positioned itself as a pioneer in responsible AI deployment across health systems.
3) How Drift improved response times in marketing chatbots
Drift is a conversational marketing platform that deploys chatbots to engage website visitors in real-time. In the process of doing so, they provided website visitors on-the-spot access to answers to their queries and thus radically improved website visitor lead generation and satisfaction rates.
They did this by fine-tuning an AI model to reduce computational complexity without losing accuracy. At the same time, they improved their load balancing algorithms, distributing requests more efficiently across servers.
This proved vital since most websites struggled to respond quickly during peak hours.
4) How Airbnb tailored custom KPIs to improve their AI model
Airbnb used machine learning for dynamic pricing, which boosted revenue and filled rooms better for hosts.
Airbnb’s dynamic pricing algorithm analyses historical booking data, competitor prices, and external factors to optimise listing prices in real time, maximising revenue for hosts while ensuring competitive rates for guests.
Airbnb’s dynamic pricing model has led to increased booking rates and revenue for hosts, with reports indicating that hosts using dynamic pricing tools earn up to 40% more compared to those who set fixed prices.
These examples show how AI and analytics can change businesses in different areas, like streaming music to booking accommodation. By using AI, startups can make their products better, improve user experiences, and stand out in a competitive market.
Conclusion
AI product analytics is changing how we manage products in 2024. Companies are now using AI to make smarter decisions and adopting tools like Phospho lets them make sense of and handle huge amounts of data quickly, giving them key insights and actions to take.
Imagine a world where data is optimized across industries and business types, from small shops to multinational corporations. We’re not quite there yet, but that future is closer than it feels.
At Phospho we’ve built our AI product analytics platform to be as accessible as possible (not restricted to developers) so teams building LLM apps have the insights they need for highly effective iteration cycles just like Spotify and Airbnb.
If you’re creating an LLM app and want to gain untapped insights from your text data, sign up here!