What purpose do fairness measures serve in ai product development? How real-time monitoring and evaluation can ensure fair outcomes:
Fairness measures in AI product development ensure unbiased outcomes across sectors like finance and healthcare. Phospho’s real-time monitoring tools help teams set fairness metrics, addressing bias while improving AI performance, user trust, and product-market fit.
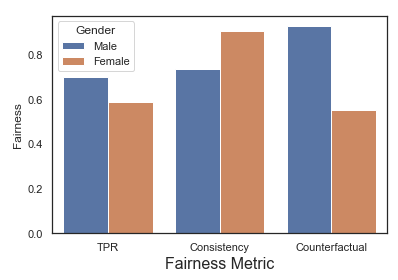
Crazy news guys, we’ve just launched a startup program for AI founders (the perks are crazy).
You can get $2000 worth of credits (Anthropic, Mistral, OpenAI, and Phospho) + a call with our amazing team to guide you in your product-market-fit journey.
You can apply here.
Understanding the Role of Fairness Measures in AI Product Development
What are fairness measures in AI?
Fairness in AI is essential to ensure that your models don’t unintentionally harm certain groups and that they work for everyone.
The way in which we can measure ‘fairness’ is through fairness metrics which help identify areas of our AI models prone to any bias that can unintentionally cause this harm.
Why fairness is essential in AI product development
Other than the obvious mistreatment of any groups or individuals, fairness is a critical component in AI systems across key sectors such as finance, healthcare, or recruitment where the livelihood of others is concerned.
The consequences beyond this also extend as far as legal risks, reputational damage, and loss of trust with users.
What purpose do fairness measures serve in AI product development?
Put simply, real-time monitoring and evaluation of such metrics during AI product development is key to ensure these fairness metrics are consistently met to promote fair outcomes and your AI models are not incurring any unnecessary risks towards your business.
The goal of this article is to show how to implement fairness measures and the tools that can let you do so easily.
How To Implement Fairness Metrics in AI Product Development
Before measuring for fairness in our AI model we first need to define our fairness metrics.
These metrics are supposed to be quantitative measures of whether our AI model actually treats individuals or groups equitably.
Examples: Defining Fairness Metrics in AI
Let’s take 3 example fairness metrics…
- Gender balance in content recommendation systems e.g job board or dating app This fairness metric can be set to measure and ensure recommendations are similar and balanced across different genders.
- Demographic parity in AI assisted law enforcement systems This metric assesses whether recommendations or predictions in criminal justice don’t disproportionately impact certain demographic groups more than others.
- Equal opportunity in the financial AI e.g loan approval or credit scoring systems This metric can help measure the proportion of positive outcomes across different groups to identify if there are any potentially discriminatory decisions being made by your AI.
To actually set these fairness metrics we need advanced tools like Phospho that let us define metrics in natural language. You can see in the next section how we can do this.
How To Implement Fairness Metrics (Step by Step)
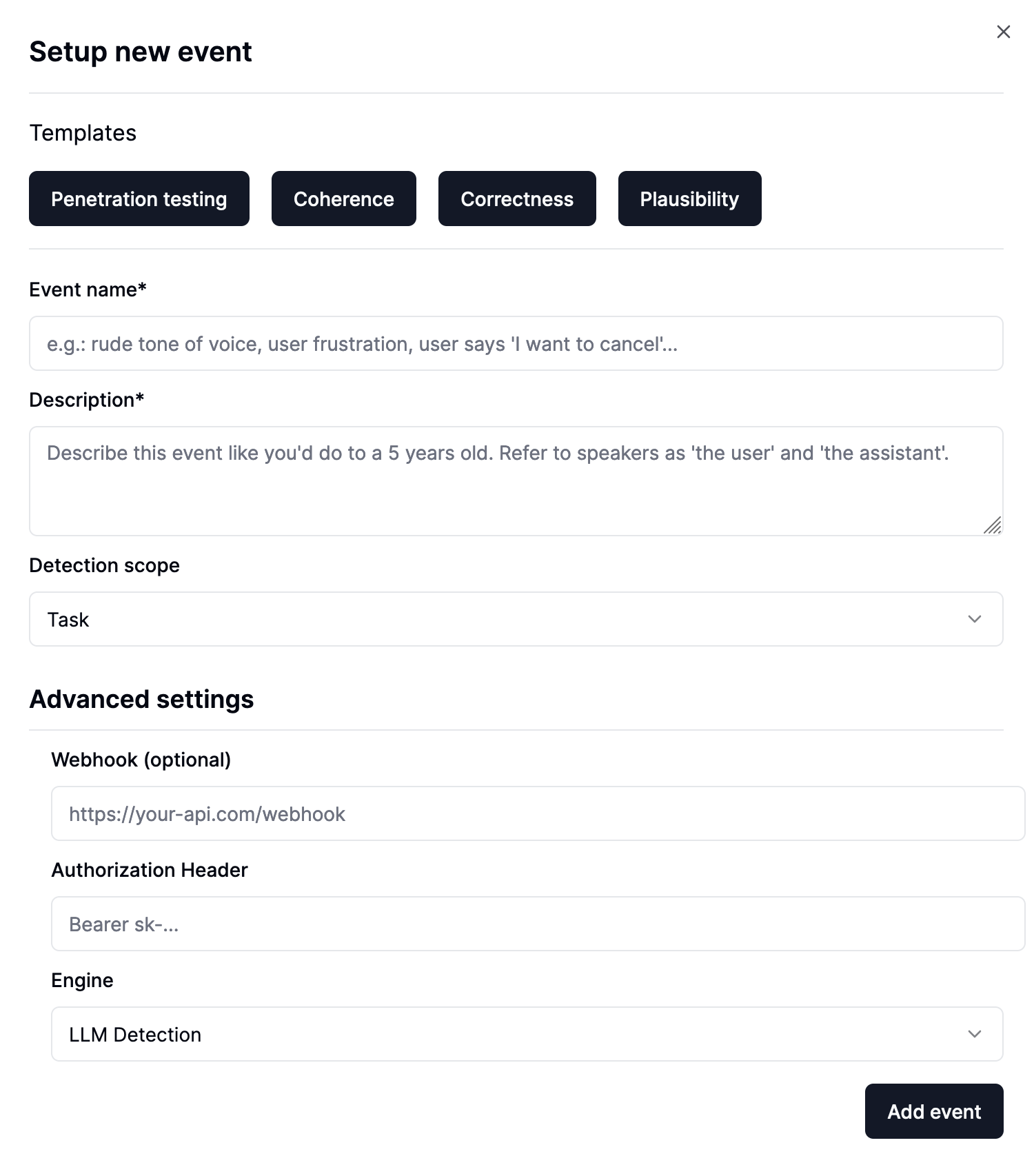
AI systems are complex and in order to both monitor and evaluate them for fairness, we cannot rely on traditional tools to identify and rectify bias in our AI models.
For that we need more advanced, AI specific tools like Phospho.
Phospho is an open source analytics tool specifically designed to monitor and evaluate the performance AI models.
In Phospho you can set custom KPIs (using natural language) to define and track any metrics with complete specificity and freedom e.g hyper specific or more broad fairness metrics.
The Process (6 Steps)
Here’s how easy the process would look with Phospho:
- Log in to Phospho and navigate to the dashboard
- Create custom tags in the analytics tab on the dashboard
- This tag definition is your custom KPI and Phospho will automatically detect and log when AI outputs meet this criteria
- Set up events or webhooks (optional): receive real-time notifications when your KPI is detected e.g Phospho notifications, Slack messages, SMS, emails etc.
- All your tags (in this case fairness metrics) are continuously monitored and can be viewed on the Phospho dashboard which can also be customised (Phospho’s custom dashboards)
Define your fairness metric in the tag definition using natural language
All that’s left after this is to monitor your dashboard and continuously refine your tag definitions (fairness metrics) based on the observed results.
You can use these insights to iteratively incorporate more fairness into your AI model.
Try Phospho for free by signing up here !
Or if you’re an AI SaaS startup you can claim $2000 worth of credits (Anthropic, Mistral, OpenAI, and Phospho) + a call with our amazing team to guide you in your product-market-fit journey.
If your company is less than two years old and has raised less than $5 million in funding, you are eligible and can apply now.
Conclusion: Why Fairness Measures, Text Analytics, and Real-Time Monitoring Are Vital for AI Product Success
Biases can emerge unexpectedly at any time so it’s vitally important that teams developing on top of AI models are constantly monitoring and evaluating user interactions in real-time.
This means teams can quickly and proactively address any identified biases or signs of unfairness before they become more problematic.
By using Phospho, you can continuously develop your AI model not just for accuracy but also for fairness to:
- build long term user trust (retention)
- ensure high AI model performance (satisfaction)
- and find product market fit faster (scale)
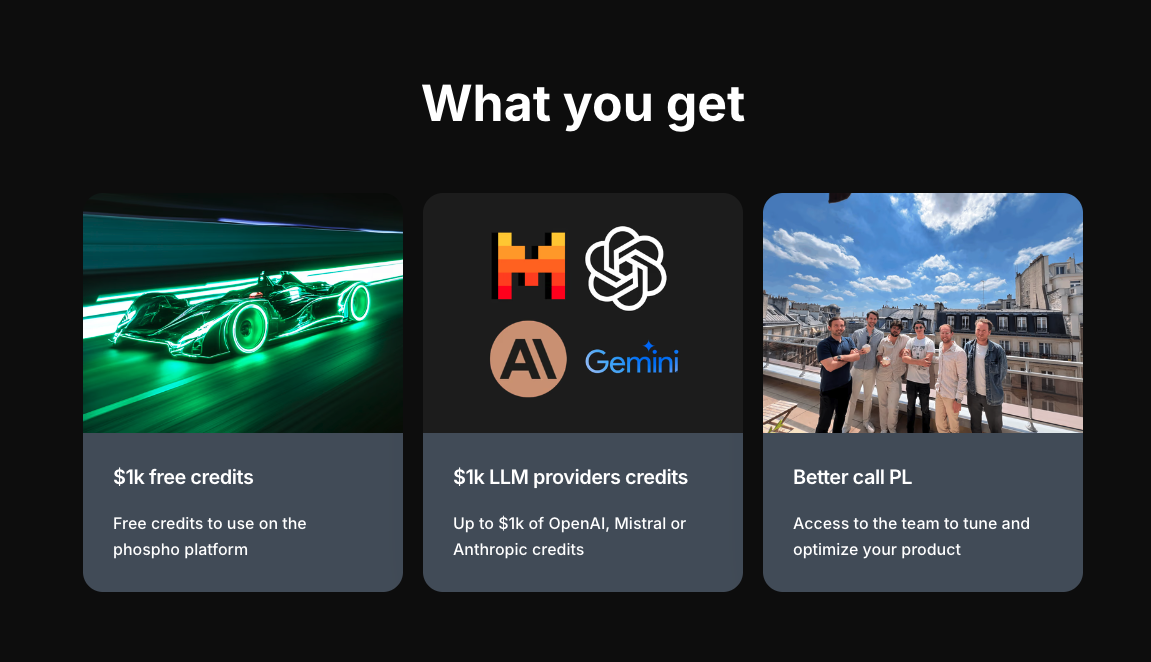
Sign up to use Phospho for free or if you’re eligible, apply to our free startup program to get $2000 of free credits towards Phospho and LLM providers!